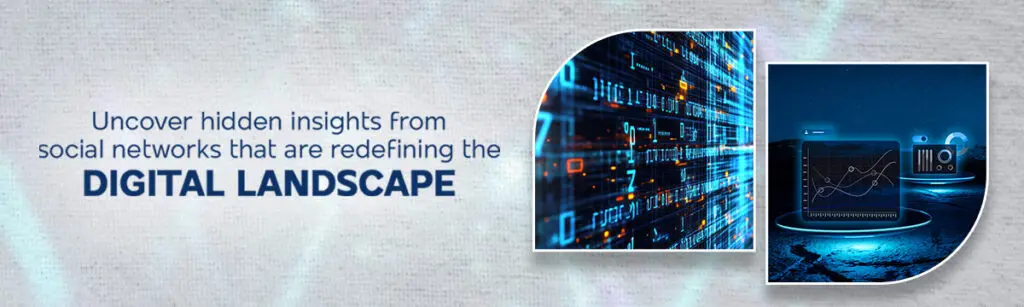
In the digital age, social networks generate an immense volume of data, offering valuable insights into user interactions, preferences, and trends. Data mining in social networks applies computational techniques to extract meaningful patterns, detect anomalies, and predict user behaviour. Understanding these methods is crucial for Computer Science Engineering students as they form the backbone of recommender systems, targeted marketing, cybersecurity, and even social influence modelling.
This blog delves into key algorithms used in big data mining, explores the identification of patterns within massive datasets, and examines how user behaviour analysis shapes technological advancements. Whether you’re interested in artificial intelligence, machine learning, or data science, mastering these concepts will open doors to innovative applications in digital ecosystems.
Key Algorithms in Social Media Data Mining
Popular social media platforms like Facebook alone process over 4 petabytes daily, making data mining essential. Data mining in social networks includes graph-based models (like PageRank) for ranking influential nodes, clustering algorithms (such as K-Means and DBSCAN) for community detection, and classification techniques (like Decision Trees and Support Vector Machines) for sentiment analysis.
Machine learning-based Natural Language Processing (NLP) techniques, including Linear Discriminant Analysis (LDA), help analyse textual data. Deep learning models, like Convolutional Neural Networks (CNNs) and Recurrent Neural Networks (RNNs), enhance image and text analytics.
These algorithms operate on parameters like engagement metrics (likes, shares, comments), user activity patterns, and network structures, enabling businesses, researchers, and policymakers to extract valuable insights from social interactions worldwide.
Pattern Recognition and Trend Analysis in Social Networks
With over 500 million postings daily and more than 4 billion interactions per day, Meta platforms rely on pattern recognition and trend analysis to process and manage vast data streams efficiently. Big data mining in social networks thrive on techniques like time-series analysis, anomaly detection, and association rule learning, help identify emerging trends, viral content, and user behaviour patterns in real-time.
Models like Hidden Markov Models (HMMs) and Long Short-Term Memory (LSTM) networks predict user engagement and content propagation, ensuring seamless platform operation. This data-driven approach prevents system congestion, enhances content recommendations, and enables proactive moderation, maintaining the fluidity and responsiveness of social networks worldwide.
User Behaviour Analysis: Insights and Applications
With 4.8 billion social media users worldwide generating an enormous data footprint, User Behaviour Analysis (UBA) is a crucial component of big data mining. Platforms like Facebook, Instagram, and Twitter rely on engagement metrics (likes, shares, dwell time, click-through rates) and predictive analytics to refine user experience and optimise content delivery.
AI-driven recommendation systems, such as those used by Netflix and YouTube, continuously analyse viewing patterns to personalise content. This process functions on a real-time feedback loop, where machine learning models track interactions, refine algorithms, and enhance engagement.
Additionally, UBA plays a critical role in fraud detection, targeted advertising, and cybersecurity, ensuring the fluidity and efficiency of social media networks across the globe while preventing data overload and operational slowdowns.
OmDayal Group of Institutions: Encouraging Prolific Computer Wizards for Big Data Mining
At our institution, we equip future tech innovators with the expertise to navigate and optimise the vast data mining in social networks. Through a robust curriculum in machine learning, artificial intelligence, and data analytics, students gain hands-on experience handling massive datasets, designing intelligent algorithms, and enhancing system efficiency.
With a strong focus on real-world applications and emerging technologies, we prepare them to tackle the challenges of big data mining, ensuring they become the next generation of problem-solvers in the ever-evolving digital ecosystem.